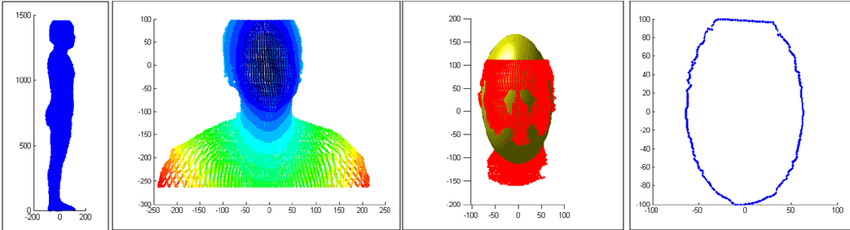
A Comparative Study of Different Face Shape Classification Techniques
Throughout the last years, there has been an increasing interest in developing useful computer vision techniques that help in many fields. Face shape classification is considered a common task in beauty and fashion purposes. The aim of this paper is to represent a comparative study of different supervised learning algorithms used in face shape classification. The classification was based on extracted facial features for the 5 different face shapes: Heart, Square, Long, Oval and Round as labels. Different classification algorithms that use landmark distance ratios and angles as features were compared: K-Nearest Neighbors (KNN), Support Vector Machine (SVM), Multilayer Perception (MLP), Random Forest (RF), AdaBoost, and Naive Bayes. Around five thousand female celebrities' images were used for training and testing the different classifiers. The results showed that the SVM classifier with radial basis function kernel achieved the highest overall accuracy of 82%. © 2021 IEEE.