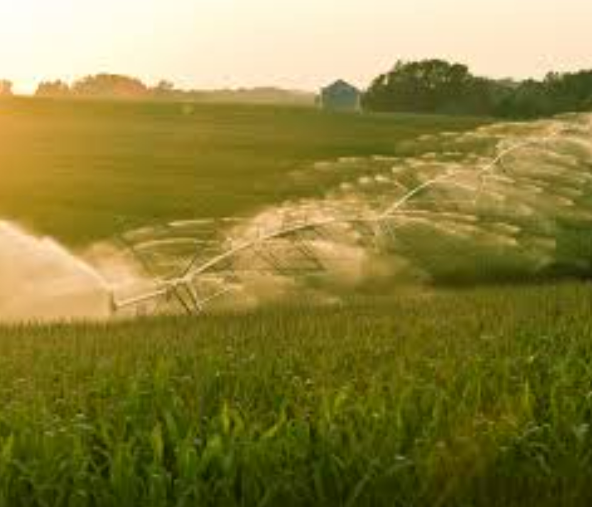
A Comparative Study of Machine Learning Techniques for Automatic Rice Crop Irrigation
Rice crop agriculture has a significant load in power systems because of the irrigation systems' huge quantity of electrical power. Thus, automation technologies are increasingly incorporating to reduce power. As a result, the costs will be reduced, and the production will be increased. This paper represents a comparative study of different supervised learning algorithms to be used in the automatic irrigation systems for the rice crop. The state of the water pump is classified based on 4 different features: soil moisture, temperature, humidity, and time. Different classification algorithms are compared: Logistic regression (LR), K-Nearest Neighbors (KNN), Decision Tree (DT), and Random Forest (RF). Around 100, 000 records are employed for the training and testing of these different models. The results reveal that the RF classifier has achieved the highest accuracy with 99.9%. © 2021 IEEE.